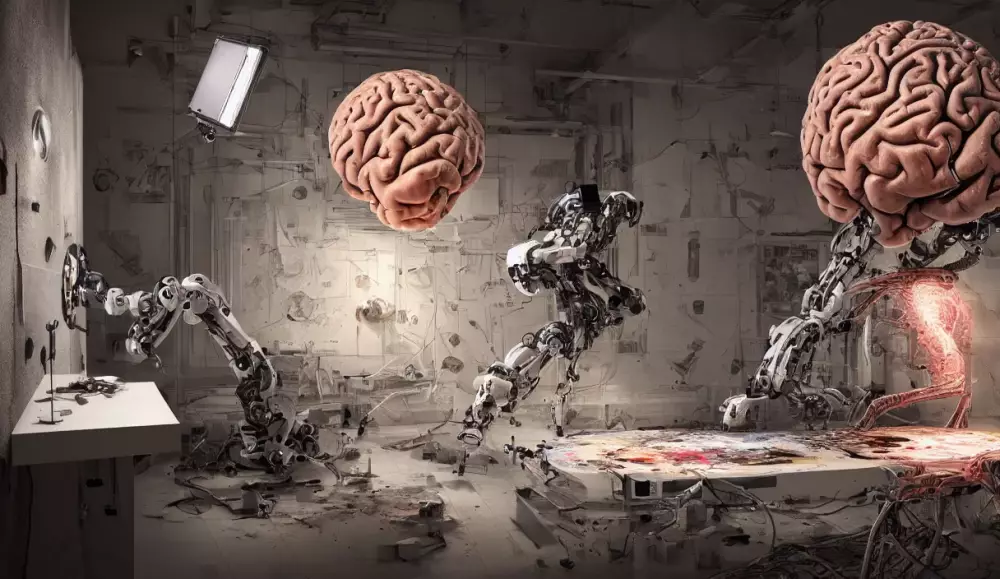
The Rise of AI-Generated Text and the Challenge of Detecting It
This sentence was written by an AI — or was it? OpenAI’s new chatbot, ChatGPT, presents us with a problem: How will we know whether what we read online is written by a human or a machine?
Since it was released in late November, ChatGPT has been used by over a million people. It has the AI community enthralled, and it is clear the internet is increasingly being flooded with AI-generated text. People are using it to come up with jokes, write children’s stories, and craft better emails.
ChatGPT is OpenAI’s spin-off of its large language model GPT-3, which generates remarkably human-sounding answers to questions that it’s asked. The magic—and danger—of these large language models lies in the illusion of correctness. The sentences they produce look right—they use the right kinds of words in the correct order. But the AI doesn’t know what any of it means. These models work by predicting the most likely next word in a sentence. They haven’t a clue whether something is correct or false, and they confidently present information as true even when it is not.
In an already polarized, politically fraught online world, these AI tools could further distort the information we consume. If they are rolled out into the real world in real products, the consequences could be devastating.
We’re in desperate need of ways to differentiate between human- and AI-written text in order to counter potential misuses of the technology, says Irene Solaiman, policy director at AI startup Hugging Face, who used to be an AI researcher at OpenAI and studied AI output detection for the release of GPT-3’s predecessor GPT-2.
New tools will also be crucial to enforcing bans on AI-generated text and code, like the one recently announced by Stack Overflow, a website where coders can ask for help. ChatGPT can confidently regurgitate answers to software problems, but it’s not foolproof. Getting code wrong can lead to buggy and broken software, which is expensive and potentially chaotic to fix.
A spokesperson for Stack Overflow says that the company’s moderators are “examining thousands of submitted community member reports via a number of tools including heuristics and detection models” but would not go into more detail.
In reality, it is incredibly difficult, and the ban is likely almost impossible to enforce.
Today’s Detection Tool Kit
There are various ways researchers have tried to detect AI-generated text. One common method is to use software to analyze different features of the text—for example, how fluently it reads, how frequently certain words appear, or whether there are patterns in punctuation or sentence length.
“If you have enough text, a really easy cue is the word ‘the’ occurs too many times,” says Daphne Ippolito, a senior research scientist at Google Brain, the company’s research unit for deep learning.
Because large language models work by predicting the next word in a sentence, they are more likely to use common words like “the,” “it,” or “is” instead of wonky, rare words. This is exactly the kind of text that automated detector systems are good at picking up, Ippolito and a team of researchers at Google found in research they published in 2019.
But Ippolito’s study also showed something interesting: the human participants tended to think this kind of “clean” text looked better and contained fewer mistakes, and thus that it must have been written by a person.
In reality, human-written text is riddled with typos and is incredibly variable, incorporating different styles and slang, while “language models very often work with clean, edited text that has been curated for machine learning purposes.” This can lead to discrepancies between how a language model and a human would approach the same text.
Additionally, language models can perpetuate biases present in the data they were trained on. For example, if a language model was trained on text that disproportionately uses masculine pronouns, it may struggle to accurately recognize and respond to text that uses gender-neutral language or includes feminine pronouns. This can lead to unintended discrimination and perpetuate existing societal biases.
Despite these limitations, language models have already proven incredibly useful in a wide range of applications, from natural language processing and sentiment analysis to machine translation and even creating entirely new content. As language models continue to advance, they are likely to become even more important in our daily lives, making it critical that we understand their limitations and work to mitigate potential negative consequences.
One of the biggest concerns regarding language models is their potential to perpetuate biases and discrimination. Since these models learn from human-written text, they can inherit the biases and prejudices that are present in our society. For example, a language model trained on text from the internet may learn to associate certain demographic groups with negative stereotypes, which could then be reflected in its output.
To address this issue, researchers are working to develop methods for detecting and mitigating bias in language models. This includes techniques such as debiasing the training data, modifying the model architecture, and post-processing the output to remove biased language.
Another concern with language models is their potential to be used for malicious purposes, such as generating fake news or impersonating individuals online. As these models become more advanced, it may become increasingly difficult to distinguish between real and fake content. This could have serious implications for democracy, privacy, and personal security.
To address these concerns, there is a need for increased transparency and accountability in the development and use of language models. This includes clear guidelines for data collection and model training, as well as regulations and oversight to ensure that language models are used ethically and responsibly.
In summary, language models have already had a significant impact on our daily lives, and their importance is likely to continue growing in the future. However, we must be aware of the potential limitations and risks associated with these models, and work to develop strategies for addressing them. By doing so, we can ensure that language models are used to benefit society as a whole, rather than contributing to harm and inequality.
Interested in the latest updates on AI technology? Follow us on Facebook and join our group (Link to Group) to leave your comments and share your thoughts on this exciting topic!